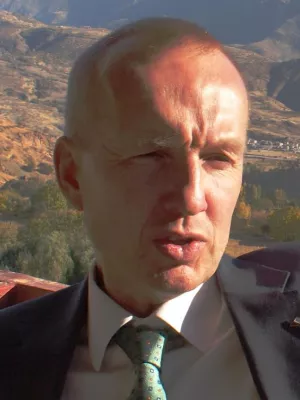
Petter Pilesjö
Professor
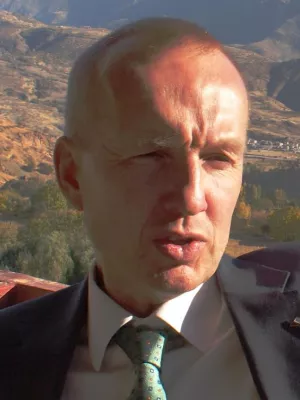
The superiority of the Adjusted Normalized Difference Snow Index (ANDSI) for mapping glaciers using Sentinel-2 multispectral satellite imagery
Author
Summary, in English
Accurate monitoring of glaciers’ extents and their dynamics is essential for improving our understanding of the impacts of climate and environmental changes in cold regions. The satellite-based Normalized Difference Snow Index (NDSI) has been widely used for mapping snow cover and glaciers around the globe. However, mapping glaciers in snow-covered areas using existing indices remains a challenging task due to their incapabilities in separating snow, glaciers, and water. This study aimed to evaluate a new satellite-based index and apply machine learning algorithms to improve the accuracy of mapping glaciers. A new index based on satellite data from Sentinel-2 was tested, which we call the Adjusted Normalized Difference Snow Index (ANDSI). ANDSI (besides NDSI) was used with five different machine learning algorithms, namely Artificial Neural Network, C5.0 Decision Tree Algorithm, Naive Bayes classifier, Support Vector Machine, and Extreme Gradient Boosting, to map glaciers, and their performance was evaluated against ground reference data. Four glacierized regions in different countries (Canada, China, Sweden, and Switzerland-Italy) were selected as study sites to evaluate the performance of the proposed ANDSI. Results showed that the proposed ANDSI outperformed the original NDSI, and the C5.0 classifier showed the best overall accuracy and Kappa among the selected five machine learning classifiers in the majority of cases. The original NDSI yielded results with an average overall accuracy of (around) 91% and the proposed ANDSI with (around) 95% for glacier mapping across all models and study regions. This study demonstrates that the proposed ANDSI serves as a superior and improved method for accurately mapping glaciers in cold regions.
Department/s
- Dept of Physical Geography and Ecosystem Science
- MECW: The Middle East in the Contemporary World
- BECC: Biodiversity and Ecosystem services in a Changing Climate
- Centre for Geographical Information Systems (GIS Centre)
- Centre for Advanced Middle Eastern Studies (CMES)
- MERGE: ModElling the Regional and Global Earth system
Publishing year
2023-09
Language
English
Publication/Series
GIScience and Remote Sensing
Volume
60
Issue
1
Document type
Journal article
Publisher
Taylor & Francis
Topic
- Remote Sensing
- Physical Geography
Keywords
- Adjusted Normalized Difference Snow Index (ANDSI)
- cold regions
- Sentinel-2
- glaciers
- machine learning classifiers
Status
Published
ISBN/ISSN/Other
- ISSN: 1548-1603